Configuring the B2C Customers Data Product
The B2C customers data product creates a mastering flow with steps specific to customer contact mastering.
This topic describes how to configure the B2C customers data product.
Adding Data to Your Data Product
Add input data to a data product using a previously uploaded source data file. To learn how to upload sources to Tamr Cloud, see Managing Source Datasets.
To add datasets, go to the Configure Data Product tab, also known as the Settings page. Select the input dropdown to add your sources. Add sources one at a time by selecting the input then Add Source.
Configuring Attributes
Automapping Attributes
Tamr quickly helps you quickly map source columns to appropriate attributes in the predefined unified schema and custom attributes, by:
- Identifying source columns that match previously mapped source columns. AutoMap applies the same mapping for the matching columns.
- Identifying source columns that match unified schema attributes. AutoMap maps these columns to their matching unified schema attributes.
When automapping, Tamr considers columns and attributes to be a match when they contain the same words, not including delimiter characters, plural words, and partial matches. Delimiters recognized include camel case, but not lower case characters. Tamr does not automatically map two columns from a single source to the same output attribute, and does not create an output attribute if no match is found for a selected column.
Two names are considered a match if the names are an exact match when split on:
- The following characters:
- \ _ ( ) / \
- The boundary between lowercase and uppercase letters.
- The boundary between letters and numbers.
- Whitespace characters.
Example | Resulting Action |
---|---|
Match cases | Match: addressLine1 , address_line_1 , and Address Line (1) These 3 columns would be mapped to the unified schema attribute address_line_1 . |
Delimiters accepted | Match: company_id and company id These 2 columns would be mapped to the unified schema attribute company_id . |
Delimiters not accepted | No match: : address|Line|1 and addressLine1 No mapping. Pipe character delimiters are not accepted. |
Plurals | No match: region and regions No mapping. |
Partials | No match: Primary Street Address and Street Address No mapping. |
Mapping Attributes
To map an input attribute from your source, you select the dropdown in your source’s column, and then choose an appropriate value. You can select the icon next to the source name to see a preview of the dataset and help you best determine how to map each attribute.
When mapping attributes, there are required, suggested, and optional attributes for you to map:
- Required: You must map source columns to these attributes. You cannot refresh your data until you do this.
- Suggested: For optimal data quality, enrichment, and clustering results, map source columns to these attributes.
- Optional: These attributes have minimal impact on your clustering and enrichment results. If your source data includes columns that match these attributes, map them to include that source data in your completed data product.
For example, in the image below, the primary key attribute must be mapped. Additionally, you can see Tamr Cloud automatically mapped source attributes to the First Name attribute.
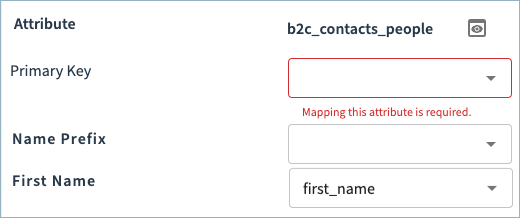
Adding Custom Attributes
At the bottom of the attribute list, you can add and map any custom attributes from your source data.
Custom attribute names must be unique and can contain only lowercase letters (a-z), numbers (0-9), and underscores (_).
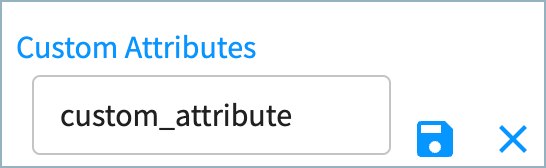
Record Consolidation Rules
Record consolidation rules create a single golden record, also called the mastered entity record, which best represents a cluster of similar source records.
You can configure rules that determine the appropriate values from the cleaned, standardized, and validated source records to include in the golden record.
Select the attribute name to set rules.
You can set attributes to be consolidated to:
- Tamr-recommended value (default). See Tamr Recommended Record Consolidation Rules for the recommended rules for each attribute.
- Distinct values. When you consolidate an attribute to its distinct values, Tamr Cloud concatenates all the attribute’s unique values into a pipe-delimited string.
- Most common value
- Longest value
In the event of a tie, the value with the least lexicographic value is selected. This means the value that comes first alphabetically is chosen. Upper case letters are of lower lexicographic value than lower case letters, so MAIN ST is of lower lexicographic value than Main St.
In addition to record consolidation rules, you can also configure golden record values using these settings:
- Use Address Enrichment Results in Golden Records setting in the Address Standardization section. By default, record consolidation rules select address values from the enhanced source records, which have been cleaned, validated, and standardized by the address data quality services. To use the original address values in the golden records instead, you can disable this setting.
- Use Enrichment Results in Golden Records setting in the Prioritize Enrichment Providers section. This option is available only if you are using Dun & Bradstreet enrichment, and allows you to choose to use D&B enrichment results as the values for specific golden record attributes.
For Address, Phone, and Email attributes, configure record consolidation rules at the group level. Select the name of the group (Primary, Office, and so on) to open the configuration rules. In the example below, the primary address attributes are consolidated to the most common primary address from the source record cluster.
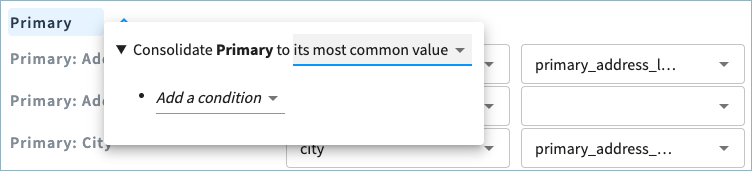
Writing Consolidation Rules
When you consolidate an attribute to its distinct values, or the most common or longest value, you can add further conditions to:
- Exclude records with empty attributes.
- Constrain records to those that include the minimum, maximum, or most common value for a specific attribute.
- Use value comparison to constrain records to those in which specified attribute values match or do not match specific values, or the values of other specified attributes.
- Prioritize records for one ore more specific source datasets.
See the below examples to understand how the following conditions work.
Exclusion Example
For example, in the image below, Middle Name is set to the most common value, with the condition to exclude any records with empty values for First Name. Records with empty values for First Name will be excluded when identifying the most common Middle Name value from the clustered source records.
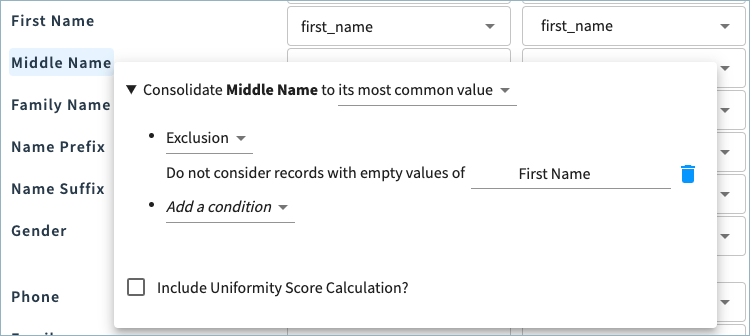
Constraint Example
You could set Middle Name to the most common value, when considering only records containing the most common First Name value.
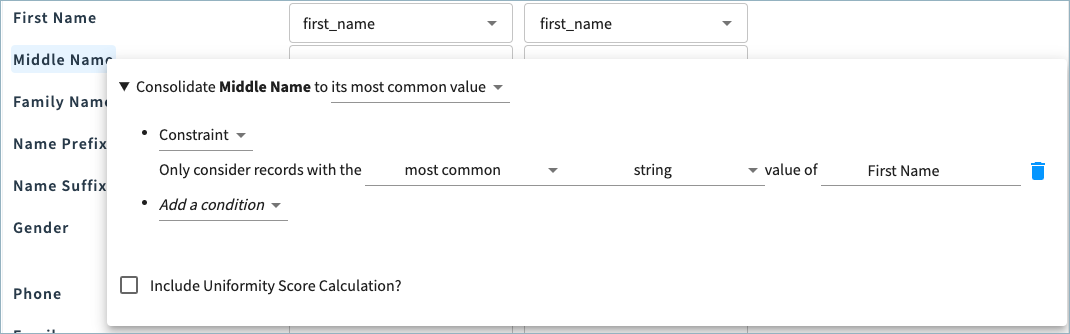
Value Comparison Example
The value comparison condition allows you to consider only records in which specified attribute values match or do not match specific values, or the values of other specified attributes.
In the example below, the data product includes a custom attribute for account_status
. Source values can be active
, inactive
, or removed
.
Full Name is set to the most common value, excluding empty values, from records in which the account_status
is active
.
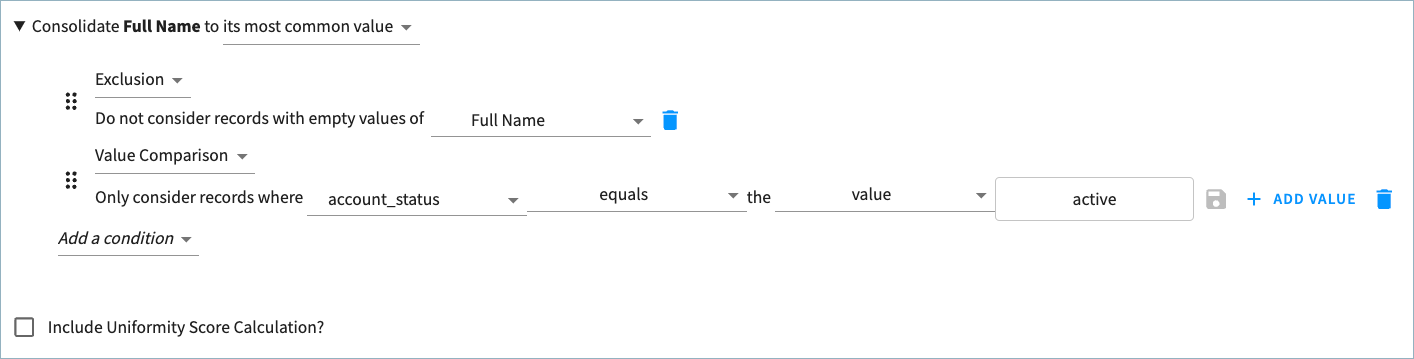
Prioritization Example
When you prioritize a dataset, if there is a tie for the most common or longest value, the attribute from the prioritized dataset is chosen. Any datasets not specified in the rule are considered lowest priority. In the image below, the Primary address group is set to the most common value, with the condition to prioritize records from the source test_data.csv
.
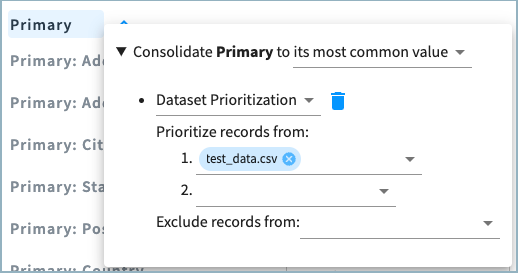
Configuring Uniformity Score
For each attribute, select whether to calculate the uniformity score. The uniformity score provides insight into how similar the values for this attribute are within the source record cluster. Uniformity scores range from 0 to 1. A uniformity score of 1 for an attribute means that all records in the cluster have the same value for this attribute, while a uniformity score of 0 indicates that all records in this cluster have different values for this attribute.
Null values are not included when calculating the attribute uniformity score. If all values for the attribute in the cluster are null, a null uniformity score is returned.
When you select to calculate the uniformity score of an attribute, a uniformity score icon appears next to the attribute name.
Additionally, Tamr automatically calculates the overall uniformity score for each cluster, which indicates how similar clustered source records are to each other. Null values are not included when calculating this score.
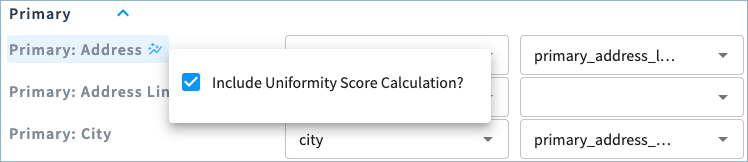
Configuring Clustering Rules
You can fine-tune clustering results by applying clustering rules. After the model has clustered source records, these rules can match or split clusters based on values in specific attributes. For example, if you trust that a user’s social security number uniquely identifies a person, you may want to create a rule that always clusters together records with matching social security numbers and does not cluster records with different social security numbers.
Clustering rules are applied only when the specified attribute values are exact, non-null, case-sensitive matches.
You can add up to three rules. See Understanding Clustering for more information on clustering.
There are three types of clustering rules:
- Match: Matches clusters with matching non-null values for the specified attributes, such as a
company_name
. Match rules will not match clusters that contain only null or empty values for the specified attribute. If you specify more than one attribute in the rule, the rule is applied only if the values for both attributes are matching and non-null. - Split: Splits clusters that contain records with different non-null values for the specific attributes. The rule splits the cluster so that each new cluster contains records with matching values for the attribute. If you specify more than one attribute in the rule, the rule is applied only if the values for both attributes are different and non-null.
- Both: For the specified attributes, matches clusters with matching non-null values and splits clusters that contain records with different non-null values, following the logic in the bullets above.
Each rule is numbered. After the data product runs, the Applied Clustering Rules (clustering_metadata.applied_clustering_rules
) attribute in the source records dataset provides the number assigned to any rules applied to the record.
Configuring Data Cleaning
To improve your data before matching, you can choose to:
- Remove known bad or placeholder values from your source records.
- Transliterate name values from non-Latin alphabets to similar-sounding characters in the Latin alphabet.
Cleansing Values
Tamr automatically replaces specific, common bad values with null
wherever it identifies an exact, case insensitive match in the mapped source record fields. See Automatically Cleaned Data Values for the values that are cleaned automatically.
Additionally, the data cleaning feature enables you to replace known bad values in your source data with null
, when Tamr finds an exact, case insensitive match for an attribute value. This helps to ensure that these values are not used for matching or included in your golden records.
The values that you specify are replaced with null
wherever they appear as values in the mapped data product schema. For example, you may want to remove values such as 555-5555
, test
, and so on.
Consider this example when the value 555-5555
is specified for data cleansing:
- If the attribute value is
555-5555
, the value is changed tonull
. - If the attribute value is
1-800-555-5555
, the full original value is retained. (It is not changed to1-800-
.)
Specify a value to be cleaned by selecting Add Value and then entering the exact string to be replaced with null
. You can easily edit or delete values.
After running the data product, the null
replacement values are included in the enhanced source records dataset and in the golden records (with the exception of address fields for which the original values are retained). See Publishing Data Products for more information about enhanced source records.
Wildcard support
You can use the following wildcards when specifying values to be cleaned:
- A percent sign (
%
) matches any number of characters. For example,%test%
matchestests
,testdata
andsometestdata
. - An underscore (
_
) matches a single character. For example,test_
matchestests
but nottestdata
.
You can escape _
or %
characters using two backslashes (for example, \\%
).
Applying Transliteration for Name Values
For first, middle, family, and full name values, you can choose whether to apply and use transliterated values for record clustering. This option is enabled by default.
Tamr utilizes the International Components for Unicode (ICU) transliteration service to convert non-Latin characters to similar-sounding characters in the Latin alphabet. Transliteration can improve matching by identifying matching names that are entered in a non-Latin alphabet in one record and a Latin alphabet in another.
See the ICU Transliterators documentation for information regarding supported alphabets and caveats around transliteration results for specific alphabets.
Configuring Address Standardization
In the Address Standardization section, you can:
- Choose whether golden records include original the source address values or enhanced source values that have been validated and standardized by the address data quality services.
- Choose whether to standardize country and state values to their ISO 3166 country and state codes.
Using Enhanced Source Record Address Values in Golden Records
The Use Address Enrichment Results in Golden Records option allows you to choose whether to use the original source values or enhanced high-precision source values for address fields in your golden records. Enhanced source values have been cleaned, validated, and standardized by the Address Standardization, Validation, and Geocoding service. This option is enabled by default.
Criteria for High Confidence Address Enrichment Results
Source address data is replaced with results from the the Address Standardization, Validation, and Geocoding service when the returned address data is matched with high precision. High-precision address matches are those in which either:
- The location type is rooftop, which indicates the address match is based on a precise geocode. (
enriched_address.<address_type>._location_type: rooftop
) - The location type is range interpolated AND the match type is single match.
- Range interpolated indicates that the address match is based on an approximation between two points, such as a road between intersections.
(enriched_address.\<address_type>.location_type: range_interpolated
) - Single match indicates that the address was matched to a single complete geocoding match. (
enriched_address.<address_type>._match_type: single_match
)
- Range interpolated indicates that the address match is based on an approximation between two points, such as a road between intersections.
For address matches at lower precision, the original source data is used in the golden records.
Using Enhanced Address Values for Address Line 2 Values
Address Line 2 values are replaced with values from enriched_address.<address_type>.sub_premise
if either:
- The source value is empty
- The source value does not include information indicating it is an apartment, building, department, floor, PO BOX, room suite, or unit.
Specifically, Address Line 2 values are not replaced with the enriched sub premise value if the source values includes any of the following (case-insensitive) values:
apartment
apt
building
bldg
department
dept
floor
po box
room
suite
ste
unit
#
Standardizing Country and State Values to ISO 3166 Codes
If you have enabled using address enrichment results in golden records, you can also choose to whether to standardize country and state names to two-character ISO 3166 codes.
Country codes are available for all countries; state codes are available only for the United States and Canada. The country and state names must be spelled correctly in order to be standardized to the ISO 3166 code.
See the ISO 3166 reference for more details about these codes.
This option is enabled by default.
Running Your Data Product and Viewing Results
To run your data product and apply all enrichment, clustering, and record consolidation rules, scroll to the top of the page and in the top right corner, select Refresh Data. Below the Refresh Data button, you can see the last time your results were refreshed.
To view your results, go to the Entities page, or go to Insights to see key metrics.
Configuring Your Data Product for Tamr RealTime
If you are using the Tamr RealTime offering with this data product, see About Tamr RealTime for instructions on configuring the data product for real-time use cases.
Updated 26 days ago